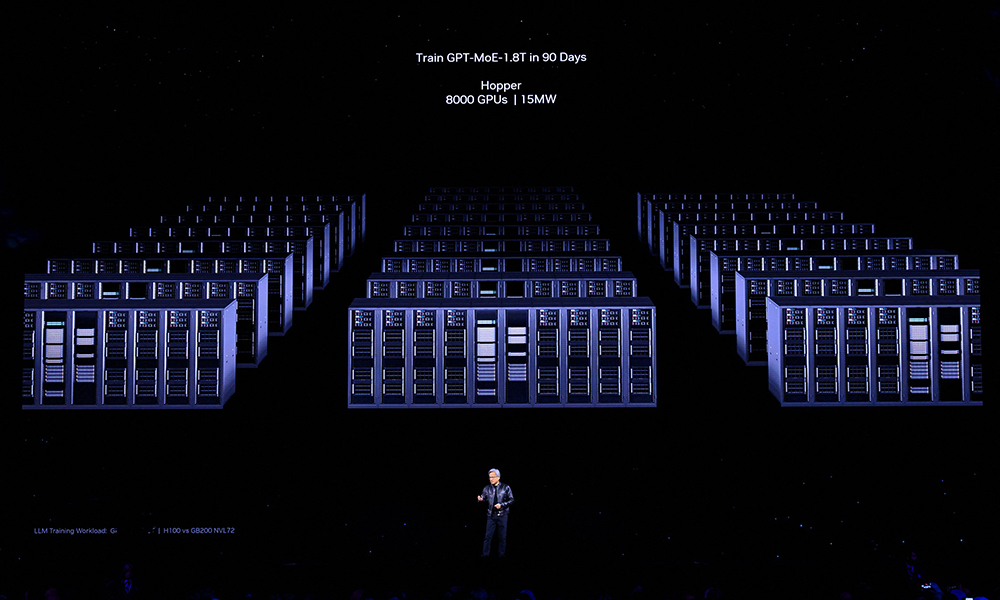
人工智能通常被視為現代社會的電力,每天為無數人類互動提供動力。然而,隨著大型科技公司和富裕國家在該領域占據主導地位,初創公司和發展中國家面臨著明顯的劣勢,尤其是在兩個關鍵領域:訓練數據集和算力。
人工智能的全球監管環境極為復雜,并因私營和公共部門利益相關者之間不同的法規和合作模式而呈分散態勢。由于需要協調國際間的監管框架和標準,這種復雜性進一步加劇。
人工智能訓練數據集的合理使用規定因地區而異。例如,歐盟的《人工智能法》禁止在未經版權所有者明確授權的情況下使用受版權保護的材料來訓練人工智能模型。相反,日本的《文本和數據挖掘法》允許在不區分合法和非法獲取的材料的情況下使用受版權保護的數據來訓練人工智能模型。相比之下,中國出臺了若干原則和法規來規范人工智能訓練數據集的使用,這些原則和法規要求合法獲取訓練數據,這一點與歐盟更為一致。然而,這些法規僅針對面向公眾的人工智能服務,而不包括企業和研究機構開發和使用的人工智能服務。
監管環境往往會塑造初創公司的發展軌跡,對其創新和規模擴張能力產生重大影響。一家專注于訓練模型的人工智能初創公司——無論是預訓練還是后訓練階段——都將面臨各種各樣的監管挑戰,這些挑戰可能會影響其長期成功,具體取決于其運營的地區。例如,在爬取受版權保護的互聯網數據并將其用于訓練強大的人工智能模型方面,日本的初創企業將受到日本《文本和數據挖掘法》的保護,這使其比歐盟的初創企業更具優勢。鑒于人工智能技術超越國界,這就需要關鍵利益攸關方開展協作,制定跨境解決方案,并進行全球合作。
就算力而言,大型企業(無論是國有企業還是私營企業)與初創企業之間存在巨大差距。大型科技公司和國有實體擁有購買和囤積算力的資源,以支持其未來的人工智能發展目標,而不具備這些資源的小型企業則依賴大型企業提供人工智能訓練和推理基礎設施。圍繞計算資源的供應鏈問題加劇了這一差距,這在全球南方更為明顯。例如,在全球前100個能夠訓練大型人工智能模型的高性能計算(HPC)集群中,沒有一個托管在發展中國家。
2023年10月,作為聯合國秘書長數字合作路線圖的一部分,聯合國高級別人工智能咨詢機構(HLAB)成立,旨在為聯合國成員國提供人工智能國際治理的分析和建議。該小組由39名具有不同背景的人士組成(按地域、性別、年齡和學科劃分),涵蓋政府、公民社會、私營部門和學術界,以確保人工智能治理的建議既公平又具有包容性。
作為這個過程的一部分,我們對初創企業和中小企業的專家進行了采訪,以探討他們在人工智能訓練數據集方面面臨的挑戰。他們的反饋意見強調了聯合國等中立國際機構在監督人工智能國際治理方面的重要性。
聯合國高級別人工智能咨詢機構關于人工智能訓練數據集標準的建議,涵蓋了預訓練還是后訓練的標準,詳見新報告《以人為本的人工智能治理》,包括以下內容:
1.建立全球匿名數據交換市場,規范數據相關定義、人工智能訓練數據全球治理原則和人工智能訓練數據來源,以及透明、基于權利的問責制。這包括引入數據管理和交換流程及標準。
2.促進數據共享,鼓勵對代表性不足或缺失的數據進行整理。
3.確保國際數據訪問的互操作性。
4.創建以尊重權利的方式補償數據創建者的機制。
為了解決算力的差距,聯合國高級別人工智能咨詢機構提出以下建議:
1.在共同利益框架下建立能力建設網絡,確保人工智能利益的公平分配。
2.建立全球基金,支持旨在將人工智能應用于當地公共利益用例的研究人員和開發人員獲取計算資源。
人工智能的國際治理,尤其是訓練數據集和算力的治理,對初創企業和發展中國家至關重要。它為獲取基本資源和促進國際合作提供了強有力的框架,使初創企業能夠在全球人工智能領域負責任地進行創新和擴展。(財富中文網)
納茲尼恩·拉賈尼(Nazneen Rajani)博士擔任Collinear AI公司的首席執行官,也是聯合國高級別人工智能咨詢機構的成員。
Fortune.com上發表的評論文章中表達的觀點,僅代表作者本人的觀點,不代表《財富》雜志的觀點和立場。
譯者:中慧言-王芳
人工智能通常被視為現代社會的電力,每天為無數人類互動提供動力。然而,隨著大型科技公司和富裕國家在該領域占據主導地位,初創公司和發展中國家面臨著明顯的劣勢,尤其是在兩個關鍵領域:訓練數據集和算力。
人工智能的全球監管環境極為復雜,并因私營和公共部門利益相關者之間不同的法規和合作模式而呈分散態勢。由于需要協調國際間的監管框架和標準,這種復雜性進一步加劇。
人工智能訓練數據集的合理使用規定因地區而異。例如,歐盟的《人工智能法》禁止在未經版權所有者明確授權的情況下使用受版權保護的材料來訓練人工智能模型。相反,日本的《文本和數據挖掘法》允許在不區分合法和非法獲取的材料的情況下使用受版權保護的數據來訓練人工智能模型。相比之下,中國出臺了若干原則和法規來規范人工智能訓練數據集的使用,這些原則和法規要求合法獲取訓練數據,這一點與歐盟更為一致。然而,這些法規僅針對面向公眾的人工智能服務,而不包括企業和研究機構開發和使用的人工智能服務。
監管環境往往會塑造初創公司的發展軌跡,對其創新和規模擴張能力產生重大影響。一家專注于訓練模型的人工智能初創公司——無論是預訓練還是后訓練階段——都將面臨各種各樣的監管挑戰,這些挑戰可能會影響其長期成功,具體取決于其運營的地區。例如,在爬取受版權保護的互聯網數據并將其用于訓練強大的人工智能模型方面,日本的初創企業將受到日本《文本和數據挖掘法》的保護,這使其比歐盟的初創企業更具優勢。鑒于人工智能技術超越國界,這就需要關鍵利益攸關方開展協作,制定跨境解決方案,并進行全球合作。
就算力而言,大型企業(無論是國有企業還是私營企業)與初創企業之間存在巨大差距。大型科技公司和國有實體擁有購買和囤積算力的資源,以支持其未來的人工智能發展目標,而不具備這些資源的小型企業則依賴大型企業提供人工智能訓練和推理基礎設施。圍繞計算資源的供應鏈問題加劇了這一差距,這在全球南方更為明顯。例如,在全球前100個能夠訓練大型人工智能模型的高性能計算(HPC)集群中,沒有一個托管在發展中國家。
2023年10月,作為聯合國秘書長數字合作路線圖的一部分,聯合國高級別人工智能咨詢機構(HLAB)成立,旨在為聯合國成員國提供人工智能國際治理的分析和建議。該小組由39名具有不同背景的人士組成(按地域、性別、年齡和學科劃分),涵蓋政府、公民社會、私營部門和學術界,以確保人工智能治理的建議既公平又具有包容性。
作為這個過程的一部分,我們對初創企業和中小企業的專家進行了采訪,以探討他們在人工智能訓練數據集方面面臨的挑戰。他們的反饋意見強調了聯合國等中立國際機構在監督人工智能國際治理方面的重要性。
聯合國高級別人工智能咨詢機構關于人工智能訓練數據集標準的建議,涵蓋了預訓練還是后訓練的標準,詳見新報告《以人為本的人工智能治理》,包括以下內容:
1.建立全球匿名數據交換市場,規范數據相關定義、人工智能訓練數據全球治理原則和人工智能訓練數據來源,以及透明、基于權利的問責制。這包括引入數據管理和交換流程及標準。
2.促進數據共享,鼓勵對代表性不足或缺失的數據進行整理。
3.確保國際數據訪問的互操作性。
4.創建以尊重權利的方式補償數據創建者的機制。
為了解決算力的差距,聯合國高級別人工智能咨詢機構提出以下建議:
1.在共同利益框架下建立能力建設網絡,確保人工智能利益的公平分配。
2.建立全球基金,支持旨在將人工智能應用于當地公共利益用例的研究人員和開發人員獲取計算資源。
人工智能的國際治理,尤其是訓練數據集和算力的治理,對初創企業和發展中國家至關重要。它為獲取基本資源和促進國際合作提供了強有力的框架,使初創企業能夠在全球人工智能領域負責任地進行創新和擴展。(財富中文網)
納茲尼恩·拉賈尼(Nazneen Rajani)博士擔任Collinear AI公司的首席執行官,也是聯合國高級別人工智能咨詢機構的成員。
Fortune.com上發表的評論文章中表達的觀點,僅代表作者本人的觀點,不代表《財富》雜志的觀點和立場。
譯者:中慧言-王芳
Artificial Intelligence (AI) is often regarded as the modern-day equivalent of electricity, powering countless human interactions daily. However, startups and developing nations face a clear disadvantage as Big Tech companies and richer nations dominate the field, especially when it comes to two critical areas: training datasets and computational power.
The global regulatory landscape for AI is highly complex and fragmented along the lines of varied regulations and collaborations between stakeholders in both the private and public sectors. This complexity is further exacerbated by the need to harmonize regulatory frameworks and standards across international borders.
The regulations governing fair use of AI training datasets differ across regions. For instance, the European Union’s AI Act prohibits the use of copyrighted materials for training AI models without explicit authorization from rights holders. Conversely, Japan’s Text and Data Mining (TDM) law permits the use of copyrighted data for AI model training, without distinguishing between legally and illegally accessed materials. In contrast, China has introduced several principles and regulations to govern the use of AI training datasets that are more in line with the EU in that they require the training data to be lawfully obtained. However, those regulations only target AI services accessible to the general public and exclude those developed and used by enterprises and research institutions.
The regulatory environment often shapes a startup’s trajectory, significantly influencing its ability to innovate and scale. An AI startup focused on training models—whether in the pre-training or post-training phase—will encounter varying regulatory challenges that could affect its long-term success, depending on the region in which it operates. For example, a startup in Japan would have an advantage over one in the EU when it comes to crawling internet data that is copyrighted and using it for training powerful AI models because it would be protected by Japan’s TDM law. Given that AI technologies transcend national borders, this necessitates collaborative, cross-border solutions, and global cooperation among key stakeholders.
In terms of computational power, a significant disparity exists between large players—whether state-owned or private entities—and startups. Bigger tech companies and state entities have the resources to buy and hoard computational power that would support their future AI development goals, whereas smaller players that do not have those resources depend on the bigger players for AI training and inference infrastructure. The supply chain issues surrounding compute resources have intensified this gap, which is even more pronounced in the global South. For example, out of the top 100 high-performance computing (HPC) clusters in the world capable of training large AI models, not one is hosted in a developing country.
In October 2023, the UN’s High-Level Advisory Body (HLAB) on AI was formed as part of the UN Secretary-General’s Roadmap for Digital Cooperation, and designed to offer UN member states analysis and recommendations for the international governance of AI. The group is made up of 39 people with diverse backgrounds (by geography, gender, age, and discipline), spanning government, civil society, the private sector, and academia to ensure recommendations for AI governance are both fair and inclusive.
As part of this process, we conducted interviews with experts from startups and small-to-medium enterprises (SMEs) to explore the challenges they face in relation to AI training datasets. Their feedback underscored the importance of a neutral, international body, such as the United Nations, in overseeing the international governance of AI.
The HLAB’s recommendations on AI training dataset standards, covering both pre-training and post-training, are detailed in the new report Governing AI for Humanity and include the following:
1.Establishing a global marketplace for the exchange of anonymized data that standardizes data-related definitions, principles for global governance of AI training data and AI training data provenance, and transparent, rights-based accountability. This includes introducing data stewardship and exchange processes and standards.
2.Promoting data commons that incentivize the curation of underrepresented or missing data.
3.Ensuring interoperability for international data access.
4.Creating mechanisms to compensate data creators in a rights-respecting manner.
To address the compute gap, the HLAB proposes the following recommendations:
1.Developing a network for capacity building under common-benefit frameworks to ensure equitable distribution of AI’s benefits.
2.Establishing a global fund to support access to computational resources for researchers and developers aiming to apply AI to local public interest use cases.
International governance of AI, particularly of training datasets and computational power, is crucial for startups and developing nations. It provides a robust framework for accessing essential resources and fosters international cooperation, positioning startups to innovate and scale responsibly in the global AI landscape.
Nazneen Rajani, PhD, is the CEO of Collinear AI and a member of the UN’s High-Level Advisory Body on AI.
The opinions expressed in Fortune.com commentary pieces are solely the views of their authors and do not necessarily reflect the opinions and beliefs of Fortune.