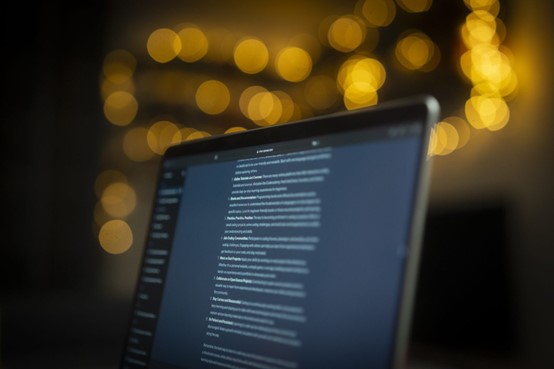
一切要從詹姆斯·鄒收到的一封郵件說起。
這封郵件提出了一個貌似合理的要求,但鄒意識到,這個要求幾乎不可能滿足。
郵件開頭寫道:“親愛的研究者:如您所知,參與者可隨時退出英國生物樣本庫(UK Biobank),并要求不得繼續使用其數據。自從我們上一次審查以來,一些參加申請[經過修改]的參與者已經要求不得繼續使用他們的數據。”
這封郵件來自英國生物樣本庫,這是一個大型健康與基因數據庫,收集了50萬英國人的數據。該數據庫被公共和私營部門廣泛應用。
鄒是斯坦福大學(Stanford University)的教授,也是一位知名生物醫學數據科學家。他已經將生物樣本庫的數據輸入到算法當中,用于訓練一個人工智能模型。現在,這封郵件要求他刪除數據。鄒在2019年舉辦的有關此事的研討會上解釋稱:“事情變得很棘手。”
這是因為,從一個經過訓練的人工智能模型中刪除一個用戶的數據幾乎是不可能的,除非將模型重置,而這樣一來為訓練模型所付出的大量金錢和精力就打了水漂。如果用人類來類比的話,人工智能“看見了”某個事物,你就很難告訴模型要“忘掉”它所看到的東西。而且徹底刪除模型也出奇地難。
這是人工智能時代初期,我們所遇到的最棘手的、尚未解決的挑戰之一,其他問題包括人工智能“幻覺”和解釋人工智能的某些輸出結果時存在的困難。許多專家認為,人工智能遺忘問題,與對隱私和虛假信息監管不足這個問題產生了沖突:隨著人工智能模型的規模日益龐大,并吸納越來越多數據,如果沒有從模型中刪除數據甚至刪除模型本身的解決方案,受影響的將不止是健康研究的參與者,這將成為一個涉及每個人的突出問題。
為什么很難徹底刪除人工智能模型
在鄒最初遭遇困境多年以后,ChatGPT等生成式人工智能工具引發的熱度,掀起了一波創作和擴散人工智能的潮流。此外,這些模型的規模越來越大,這意味著它們在訓練過程中吸收了更多數據。
許多模型被用于醫療、金融等行業,在這些行業重視數據隱私和數據使用尤為重要。
但正如鄒最初所發現的情況一樣,從模型中刪除數據并不容易。這是因為人工智能模型不止是一行行的代碼。它是經過學習后掌握的在特定數據集中不同數據點之間的統計學關系,其中包含了人類難以理解的極其復雜的微妙關系。一旦模型學會這些關系,就很難讓其忽視它已經學會的某些部分。
紐約大學(New York University)的人工智能專家和計算機科學教授阿納斯·巴里對《財富》雜志表示:“如果一個基于機器學習的系統已經接受過數據訓練,要追溯性刪除部分數據的唯一方法就是從零開始重新訓練算法。”
這個問題不止關于個人數據隱私。如果一個人工智能模型被發現收集了存在偏見或惡意的數據,例如來自種族主義者的社交媒體帖子中的數據,要清理這些不良數據難度極大。
訓練或重新訓練人工智能模型成本高昂。尤其是訓練超大型“基礎模型”需要花費巨額成本,這類模型為當前生成式人工智能的蓬勃發展提供了動力。據報道,OpenAI公司CEO山姆·阿爾特曼曾表示,訓練GPT-4的成本超過1億美元。GPT-4是驅動GhatGPT高端版本的大語言模型。
這就是為什么開發人工智能模型的公司,會害怕美國聯邦貿易委員會處罰違反美國貿易法的公司時用到的一個強大工具。這個工具名為“算法追繳”。該法律程序旨在強制違法公司徹底刪除違規的人工智能模型,作為對公司的處罰。聯邦貿易委員會很少動用這項權力,通常針對的是濫用數據的公司。一個著名案例是聯邦貿易委員會對Everalbum這家公司行使了這項權力,因為該公司未經許可使用人們的生物識別數據訓練了一個面部識別系統。
但巴里表示,算法追繳假設創建人工智能系統的公司可以識別一個數據集中非法收集的部分,但事實上并非如此。數據很容易在互聯網上四處傳播,而且越來越多數據未經許可從原始來源被“抓取”,這給確定數據的原始所有權帶來了挑戰。
算法追繳存在的另外一個問題是,在實踐中,徹底刪除人工智能模型,可能像消滅僵尸一樣困難。
人工智能專家蘭斯·埃利奧特通過電子郵件對《財富》雜志表示:“試圖刪除一個人工智能模型,或許看起來很容易,似乎只要按下刪除鍵就能徹底解決問題,但實際情況并非如此。”
埃利奧特寫道,人工智能模型被刪除后很容易復原,因為可能還有模型的其他數字拷貝存在,很容易復原。
鄒表示,對于目前的狀況,要么對技術進行大幅調整,使公司遵守法律,要么立法者重新制定法規,并重新思考如何讓公司遵守規定。
創建小模型有利于保護隱私
鄒和他的合作伙伴在研究中確實提出了在不破壞整個模型的前提下,從基于聚類的簡單機器學習模型中刪除數據的一些方法。但這些方法不適用于更復雜的模型,例如支撐當前生成式人工智能繁榮發展的大多數深度學習模型。鄒和他的合作伙伴在2019年發表的一篇研究論文中建議,這些更復雜的模型可能在最開始就需要使用一種不同訓練機制,才能在不影響整個模型運行也不需要重新訓練整個模型的情況下,刪除模型中的特定統計路徑。
如果公司擔心要求其依據規定刪除用戶數據,例如歐洲多項數據隱私法律中都有這樣的規定,他們或許需要采用其他方法。事實上,至少有一家人工智能公司的業務就是完全圍繞這種觀念展開的。
德國公司Xayn從事私人個性化人工智能搜索和推薦技術研發。該公司的技術使用一個基礎模型,為每一位用戶單獨訓練一個小模型。這樣一來,該公司很容易就能根據用戶的要求刪除用戶個人的模型。
Xayn CEO兼聯合創始人列夫-尼森·倫德班克表示:“我們絕不會遇到將用戶數據輸入一個大模型的問題。”
倫德班克表示,他認為Xayn獨立的人工智能小模型比OpenAI、谷歌(Google)、Anthropic、Inflection等公司開發的龐大的大語言模型,在開發符合數據隱私規定的人工智能方面更可行。龐大的模型從互聯網中抓取了海量數據,包括個人信息,以至于公司自己通常都不能準確了解其訓練數據集中包含了哪些數據。而且,倫德班克表示,這些龐大的模型需要巨額的訓練和維護成本。
他表示,目前隱私公司和人工智能公司處在一種并行發展的狀態。
另外一家人工智能公司SpotLab試圖填補隱私與人工智能之間的空白。該公司致力于開發臨床研究模型。其創始人兼CEO米格爾·盧恩格-奧羅斯曾是一名聯合國的研究員和首席科學家。他表示,在研究人工智能的20年間,他經常思考這個缺失的環節:人工智能系統的遺忘能力。
他表示,在這方面之所以鮮有進展,原因之一是,到目前為止,沒有任何數據隱私法能迫使公司和研究人員必須認真解決這個問題。歐洲在這方面已經有所轉變,但美國仍然缺少要求公司為刪除用戶個人數據提供便利的規定。
立法者到目前為止在這方面毫無作為,因此有人希望法院能夠介入。最近有一項訴訟指控OpenAI盜用“數以百萬計美國人的”數據訓練ChatGPT模型。
而且有跡象表明,一些大型科技公司可能開始認真對待這個問題。6月,谷歌宣布發起一項研究競賽,邀請研究人員解決人工智能無法遺忘的問題。
但在這些工作取得任何進展之前,用戶的數據將繼續在日益龐大的人工智能模型中傳播,很容易成為可疑甚至危險行為針對的對象。
倫德班克表示:“我認為這是很危險的。如果有人能夠獲取這些數據,例如某些情報機構或者其他國家,這些數據很有可能被惡意利用。”(財富中文網)
翻譯:劉進龍
審校:汪皓
一切要從詹姆斯·鄒收到的一封郵件說起。
這封郵件提出了一個貌似合理的要求,但鄒意識到,這個要求幾乎不可能滿足。
郵件開頭寫道:“親愛的研究者:如您所知,參與者可隨時退出英國生物樣本庫(UK Biobank),并要求不得繼續使用其數據。自從我們上一次審查以來,一些參加申請[經過修改]的參與者已經要求不得繼續使用他們的數據。”
這封郵件來自英國生物樣本庫,這是一個大型健康與基因數據庫,收集了50萬英國人的數據。該數據庫被公共和私營部門廣泛應用。
鄒是斯坦福大學(Stanford University)的教授,也是一位知名生物醫學數據科學家。他已經將生物樣本庫的數據輸入到算法當中,用于訓練一個人工智能模型。現在,這封郵件要求他刪除數據。鄒在2019年舉辦的有關此事的研討會上解釋稱:“事情變得很棘手。”
這是因為,從一個經過訓練的人工智能模型中刪除一個用戶的數據幾乎是不可能的,除非將模型重置,而這樣一來為訓練模型所付出的大量金錢和精力就打了水漂。如果用人類來類比的話,人工智能“看見了”某個事物,你就很難告訴模型要“忘掉”它所看到的東西。而且徹底刪除模型也出奇地難。
這是人工智能時代初期,我們所遇到的最棘手的、尚未解決的挑戰之一,其他問題包括人工智能“幻覺”和解釋人工智能的某些輸出結果時存在的困難。許多專家認為,人工智能遺忘問題,與對隱私和虛假信息監管不足這個問題產生了沖突:隨著人工智能模型的規模日益龐大,并吸納越來越多數據,如果沒有從模型中刪除數據甚至刪除模型本身的解決方案,受影響的將不止是健康研究的參與者,這將成為一個涉及每個人的突出問題。
為什么很難徹底刪除人工智能模型
在鄒最初遭遇困境多年以后,ChatGPT等生成式人工智能工具引發的熱度,掀起了一波創作和擴散人工智能的潮流。此外,這些模型的規模越來越大,這意味著它們在訓練過程中吸收了更多數據。
許多模型被用于醫療、金融等行業,在這些行業重視數據隱私和數據使用尤為重要。
但正如鄒最初所發現的情況一樣,從模型中刪除數據并不容易。這是因為人工智能模型不止是一行行的代碼。它是經過學習后掌握的在特定數據集中不同數據點之間的統計學關系,其中包含了人類難以理解的極其復雜的微妙關系。一旦模型學會這些關系,就很難讓其忽視它已經學會的某些部分。
紐約大學(New York University)的人工智能專家和計算機科學教授阿納斯·巴里對《財富》雜志表示:“如果一個基于機器學習的系統已經接受過數據訓練,要追溯性刪除部分數據的唯一方法就是從零開始重新訓練算法。”
這個問題不止關于個人數據隱私。如果一個人工智能模型被發現收集了存在偏見或惡意的數據,例如來自種族主義者的社交媒體帖子中的數據,要清理這些不良數據難度極大。
訓練或重新訓練人工智能模型成本高昂。尤其是訓練超大型“基礎模型”需要花費巨額成本,這類模型為當前生成式人工智能的蓬勃發展提供了動力。據報道,OpenAI公司CEO山姆·阿爾特曼曾表示,訓練GPT-4的成本超過1億美元。GPT-4是驅動GhatGPT高端版本的大語言模型。
這就是為什么開發人工智能模型的公司,會害怕美國聯邦貿易委員會處罰違反美國貿易法的公司時用到的一個強大工具。這個工具名為“算法追繳”。該法律程序旨在強制違法公司徹底刪除違規的人工智能模型,作為對公司的處罰。聯邦貿易委員會很少動用這項權力,通常針對的是濫用數據的公司。一個著名案例是聯邦貿易委員會對Everalbum這家公司行使了這項權力,因為該公司未經許可使用人們的生物識別數據訓練了一個面部識別系統。
但巴里表示,算法追繳假設創建人工智能系統的公司可以識別一個數據集中非法收集的部分,但事實上并非如此。數據很容易在互聯網上四處傳播,而且越來越多數據未經許可從原始來源被“抓取”,這給確定數據的原始所有權帶來了挑戰。
算法追繳存在的另外一個問題是,在實踐中,徹底刪除人工智能模型,可能像消滅僵尸一樣困難。
人工智能專家蘭斯·埃利奧特通過電子郵件對《財富》雜志表示:“試圖刪除一個人工智能模型,或許看起來很容易,似乎只要按下刪除鍵就能徹底解決問題,但實際情況并非如此。”
埃利奧特寫道,人工智能模型被刪除后很容易復原,因為可能還有模型的其他數字拷貝存在,很容易復原。
鄒表示,對于目前的狀況,要么對技術進行大幅調整,使公司遵守法律,要么立法者重新制定法規,并重新思考如何讓公司遵守規定。
創建小模型有利于保護隱私
鄒和他的合作伙伴在研究中確實提出了在不破壞整個模型的前提下,從基于聚類的簡單機器學習模型中刪除數據的一些方法。但這些方法不適用于更復雜的模型,例如支撐當前生成式人工智能繁榮發展的大多數深度學習模型。鄒和他的合作伙伴在2019年發表的一篇研究論文中建議,這些更復雜的模型可能在最開始就需要使用一種不同訓練機制,才能在不影響整個模型運行也不需要重新訓練整個模型的情況下,刪除模型中的特定統計路徑。
如果公司擔心要求其依據規定刪除用戶數據,例如歐洲多項數據隱私法律中都有這樣的規定,他們或許需要采用其他方法。事實上,至少有一家人工智能公司的業務就是完全圍繞這種觀念展開的。
德國公司Xayn從事私人個性化人工智能搜索和推薦技術研發。該公司的技術使用一個基礎模型,為每一位用戶單獨訓練一個小模型。這樣一來,該公司很容易就能根據用戶的要求刪除用戶個人的模型。
Xayn CEO兼聯合創始人列夫-尼森·倫德班克表示:“我們絕不會遇到將用戶數據輸入一個大模型的問題。”
倫德班克表示,他認為Xayn獨立的人工智能小模型比OpenAI、谷歌(Google)、Anthropic、Inflection等公司開發的龐大的大語言模型,在開發符合數據隱私規定的人工智能方面更可行。龐大的模型從互聯網中抓取了海量數據,包括個人信息,以至于公司自己通常都不能準確了解其訓練數據集中包含了哪些數據。而且,倫德班克表示,這些龐大的模型需要巨額的訓練和維護成本。
他表示,目前隱私公司和人工智能公司處在一種并行發展的狀態。
另外一家人工智能公司SpotLab試圖填補隱私與人工智能之間的空白。該公司致力于開發臨床研究模型。其創始人兼CEO米格爾·盧恩格-奧羅斯曾是一名聯合國的研究員和首席科學家。他表示,在研究人工智能的20年間,他經常思考這個缺失的環節:人工智能系統的遺忘能力。
他表示,在這方面之所以鮮有進展,原因之一是,到目前為止,沒有任何數據隱私法能迫使公司和研究人員必須認真解決這個問題。歐洲在這方面已經有所轉變,但美國仍然缺少要求公司為刪除用戶個人數據提供便利的規定。
立法者到目前為止在這方面毫無作為,因此有人希望法院能夠介入。最近有一項訴訟指控OpenAI盜用“數以百萬計美國人的”數據訓練ChatGPT模型。
而且有跡象表明,一些大型科技公司可能開始認真對待這個問題。6月,谷歌宣布發起一項研究競賽,邀請研究人員解決人工智能無法遺忘的問題。
但在這些工作取得任何進展之前,用戶的數據將繼續在日益龐大的人工智能模型中傳播,很容易成為可疑甚至危險行為針對的對象。
倫德班克表示:“我認為這是很危險的。如果有人能夠獲取這些數據,例如某些情報機構或者其他國家,這些數據很有可能被惡意利用。”(財富中文網)
翻譯:劉進龍
審校:汪皓
It all started with an email James Zou received.
The email was making a request that seemed reasonable, but which Zou realized would be nearly impossible to fulfill.
“Dear Researcher,” the email began. “As you are aware, participants are free to withdraw from the UK Biobank at any time and request that their data no longer be used. Since our last review, some participants involved with Application [REDACTED] have requested that their data should longer be used.”
The email was from the U.K. Biobank, a large-scale database of health and genetic data drawn from 500,000 British residents, that is widely available to the public and private sector.
Zou, a professor at Stanford University and prominent biomedical data scientist, had already fed the Biobank’s data to an algorithm and used it to train an A.I. model. Now, the email was requesting the data’s removal. “Here’s where it gets hairy,” Zou explained in a 2019 seminar he gave on the matter.
That’s because, as it turns out, it’s nearly impossible to remove a user’s data from a trained A.I. model without resetting the model and forfeiting the extensive money and effort put into training it. To use a human analogy, once an A.I. has “seen” something, there is no easy way to tell the model to “forget” what it saw. And deleting the model entirely is also surprisingly difficult.
This represents one of the thorniest, unresolved, challenges of our incipient artificial intelligence era, alongside issues like A.I. “hallucinations” and the difficulties of explaining certain A.I. outputs. According to many experts, the A.I. unlearning problem is on a collision course with inadequate regulations around privacy and misinformation: As A.I. models get larger and hoover up ever more data, without solutions to delete data from a model — and potentially delete the model itself — the people affected won’t just be those who have participated in a health study, it’ll be a salient problem for everyone.
Why A.I. models are as difficult to kill as a zombie
In the years since Zou’s initial predicament, the excitement over generative A.I. tools like ChatGPT has caused a boom in the creation and proliferation of A.I. models. What’s more, those models are getting bigger, meaning they ingest more data during their training.
Many of these models are being put to work in industries like medical care and finance where it’s especially important to be careful about data privacy and data usage.
But as Zou discovered when he set out to find a solution to removing data, there’s no simple way to do it. That’s because an A.I. model isn’t just lines of coding. It’s a learned set of statistical relations between points in a particular dataset, encompassing subtle relationships that are often far too complex for human understanding. Once the model learns this relationship, there’s no simple way to get the model to ignore some portion of what it has learned.
“If a machine learning-based system has been trained on data, the only way to retroactively remove a portion of that data is by re-training the algorithms from scratch,” Anasse Bari, an A.I. expert and computer science professor at New York University, told Fortune.
The problem goes beyond private data. If an A.I. model is discovered to have gleaned biased or toxic data, say from racist social media posts, weeding out the bad data will be tricky.
Training or retraining an A.I. model is expensive. This is particularly true for the ultra-large “foundation models” that are currently powering the boom in generative A.I. Sam Altman, the CEO of OpenAI, has reportedly said that GPT-4, the large language model that powers its premium version of ChatGPT, cost in excess of $100 million to train.
That’s why, to companies developing A.I. models, a powerful tool that the U.S. Federal Trade Commission has to punish companies it finds have violated U.S. trade laws is scary. The tool is called “algorithmic disgorgement.” It’s a legal process that penalizes the law-breaking company by forcing it to delete an offending A.I. model in its entirety. The FTC has only used that power a handful of times, typically directed at companies who have misused data. One well known case where the FTC did use this power is against a company called Everalbum, which trained a facial recognition system using people’s biometric data without their permission.
But Bari says that algorithmic disgorgement assumes those creating A.I. systems can even identify which part of a dataset was illegally collected, which is sometimes not the case. Data easily traverses various internet locations, and is increasingly “scraped” from its original source without permission, making it challenging to determine its original ownership.
Another problem with algorithmic disgorgement is that, in practice, A.I. models can be as difficult to kill as zombies.
“Trying to delete an AI model might seem exceedingly simple, namely just press a delete button and the matter is entirely concluded, but that’s not how things work in the real world,” Lance Elliot, an A.I. expert, told Fortune in an email.
A.I. models can be easily reinstated after deletion because it’s likely other digital copies of the model exist and can be easily reinstated, Elliot writes.
Zou says that, the way things stand, either the technology needs to change substantially so that companies can comply with the law, or lawmakers need to rethink the regulations and how they can make companies comply.
Building smaller models is good for privacy
In his research, Zou and his collaborators did come up with some ways that data can be deleted from simple machine learning models that are based on a technique known as clustering without compromising the entire model. But those same methods won’t work for more complex models such as most of the deep learning systems that underpin today’s generative A.I. boom. For these models, a different kind of training regime may have to be used in the first place to make it possible to delete certain statistical pathways in the model without compromising the whole model’s performance or requiring the entire model to be retrained, Zou and his co-authors suggested in a 2019 research paper.
For companies worried about the requirement that they be able to delete users data upon request, which is a part of several European data privacy laws, other methods may be needed. In fact, there’s at least one A.I. company that has built its entire business around this idea.
Xayn is a German company that makes private, personalized A.I. search and recommendation technology. Xayn’s technology works by using a base model and then training a separate small model for each user. That makes it very easy to delete any of these individual users’ models upon request.
“This problem of your data floating into the big model never happens with us,” Leif-Nissen Lundb?k, the CEO and co-founder of Xayn, said.
Lundb?k said he thinks Xayn’s small, individual A.I. models represent a more viable way to create A.I. in a way that can comply with data privacy requirements than the massive large language models being built by companies such as OpenAI, Google, Anthropic, Inflection, and others. Those models suck up vast amounts of data from the internet, including personal information—so much that the companies themselves often have poor insight into exactly what data is contained in the training set. And these massive models are extremely expensive to train and maintain, Lundbaek said.
Privacy and artificial intelligence businesses are currently a sort of parallel development, he said.
Another A.I. company trying to bridge the gap between privacy and A.I. is SpotLab, which builds models for clinical research. Its founder and CEO Miguel Luengo-Oroz previously worked at the United Nations as a researcher and chief data scientist. In 20 years of studying A.I., he says he has often thought about this missing piece: an A.I.’s system’s ability to unlearn.
He says that one reason little progress has been made on the issue is that, until recently, there was no data privacy regulation forcing companies and researchers to expend serious effort to address it. That has changed recently in Europe, but in the U.S., rules that would require companies to make it easy to delete people’s data are still absent.
Some people are hoping the courts will step in where lawmakers have so far failed. One recent lawsuit alleges OpenAI stole “millions of Americans'” data to train ChatGPT’s model.
And there are signs that some big tech companies may be starting to think harder about the problem. In June, Google announced a competition for researchers to come up with solutions to A.I.’s inability to forget.
But until more progress is made, user data will continue to float around in an expanding constellation of A.I models, leaving it vulnerable to dubious, or even threatening, actions.
“I think it’s dangerous and if someone got access to this data, let’s say, some kind of intelligence agencies or even other countries, I mean, I think it can be really be used in a bad way,” Lundb?k said.