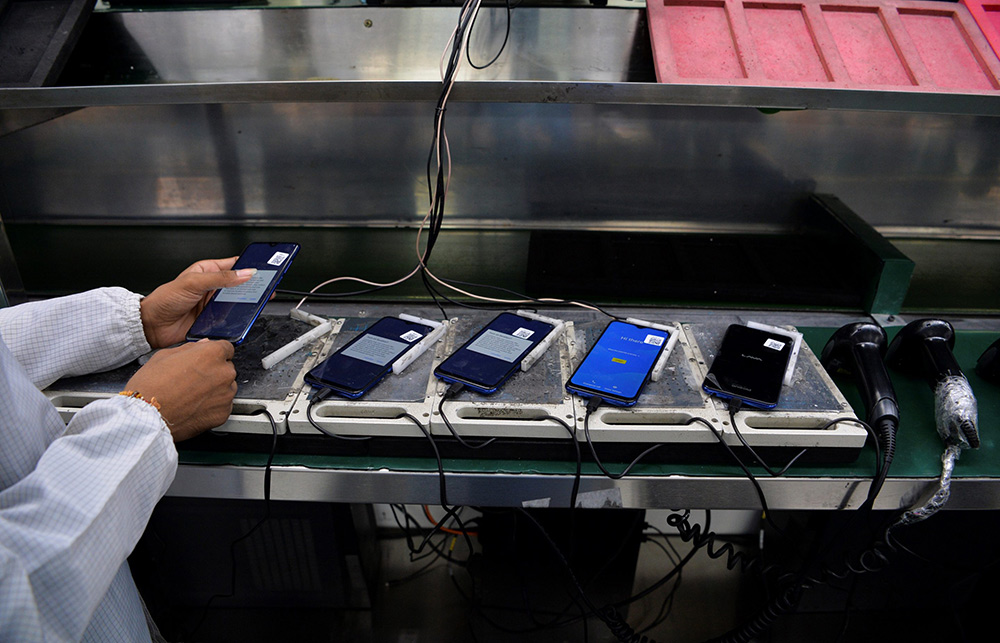
眼下是一個人人都在談論人工智能的時代,但是要想把我們新聞里看到的那些似乎很有前景的試驗轉化成有實用價值的應用,卻還是得先搞定一些很重要的工作。大型的消費型互聯網公司是第一批實際應用人工智能的企業,但他們的程序未必適合其他行業,尤其是在很多行業中,人工智能項目都面臨著一些獨特的挑戰。
因此,我們經常會看到一些非數字型企業在部署人工智能項目時遇到困難的情況。比如制造業已經做好了向人工智轉型的準備,不過MAPI基金曾對美國200多家制造業企業進行過調查,其中只有5%的受訪企業制定了清晰的人工智能戰略。埃森哲公司也曾對16個行業的1500多名高管進行過調查,有76%的受訪者表示,他們在如何促進人工智能技術的規模化應用上遇到了困難。這種現象與消費型互聯網產業形成了對比,因為在這個產業中,大型的人工智能系統已經被應用到了從生成搜索結果,到語言翻譯,再到定向廣告的各個領域。
要想充分發揮人工智能技術的潛力,人工智能技術的開發者就必須研發一些新技術,確保人工智能在所有產業得到應用。(我的公司Landing AI就是一家專門幫助企業采用人工智能技術的公司。)尤其是對于硅谷以外的企業來說,他們必須要克服三個挑戰,才能增加成功的幾率。
首先,他們必須學會利用“小數據”。一些大型科技公司會使用從幾十億用戶那里收集到的海量數據來對人工智能模型進行訓練。但是很多行業沒有那么多的數據量,所以這些針對“大數據”研發的系統必須相應地做出一些調整,以適應這些行業的“小數據”。
比如有些工廠需要利用人工智能系統,檢測智能手機表面的劃痕。沒有哪家手機廠商會有100萬部有劃痕的手機放在那里,讓人工智能系統學習劃痕長什么樣子。所以說,很多制造企業是沒有足夠的數據來支撐人工智能模型的。制造業的人工智能軟件開發者,很多時候只能拿到不到100張的樣本照片來學習。
好在一種新的“小數據”技術正在使這一切成為可能。比如現在有了一種新的數據生成技術,它只需要10張高質量的瑕疵圖片,就可以合成1000張瑕疵圖片,供人工智能系統進行學習。除此之外還有一種方法,我們可以準備1萬張來自其他產品和數據來源的瑕疵圖片,人工智能模型先學習如何從這1萬張圖片中找出瑕疵品,了解了瑕疵品的一般規律后,它就可以把這一知識應用到一種新產品上,這時只需要額外的少量瑕疵圖片,它就可以識別出這種新產品的瑕疵了。
有了這種先進的“小數據”技術,人工智能很有可以最終得以打入制造業、農業和醫療等傳統行業。
其次,實驗室里的設定與真實世界是有很大差距的,如果人工智能技術的服務對象是非數字型企業,那么就要格外重視彌合這種差距。很多人工智能系統在研究論文里或者在概念驗證中展現了很高的準確性,但在真實世界部署時,其表現就沒有這么好了。
比如說,很多研究機構都發表論文稱,人工智能技術可以通過X光或者其他醫學影像來論斷病情,準確度堪比甚至超過了職業的放射科醫師。那么,為什么直到現在,各個醫院還是很少使用這項技術呢?
其中的一個原因是,很多此類研究的基礎設定都被控制得很好,人工智能模型的學習和測試對象都是高質量的數據。人工智能模型在這樣的設定中表現得很好,就足以做概念驗證或者發表論文了。不過如果同樣的一套人工智能系統被部署在了一家真正的醫院里,只要這家醫院的X光影像稍微模糊一點,或者它的圖像采集方案稍微有一些不一樣,這套系統就適應不了了。
對于這種情況,有一種解決方案是,一開始只用人工智能系統來分析它信心度比較高的圖像,其他情況都由人類放射科醫生來解決。然后讓人工智能系統向人類醫生學習,從而逐漸承擔更多的職能。
第三,對于一些非科技企業來說,他們有必要意識到,人工智能系統的部署,很可能影響到一些員工、客戶以及其他利益相關者的利益,所以必須妥善應對好這項新技術帶來的改變。
比如說,有一種人工智能系統是專門用來幫醫生對急診室的病人進行分類的,它的應用可能會影響到很多人——比如醫生、護士乃至保險業者。為了讓項目保持正軌,人們必須積極參與人工智能技術的部署當中。而為了充分利用這項新技術,他們的工作流程也必須做出相應的調整。
我發現,很多人工智能團隊都低估了組織變革管理中的人性化的一面。要想克服這個挑戰,并不是一件容易的事。但企業卻可以采取一些措施來減輕這種影響。
首先,企業必須認識到在變革的過程中,有哪些利益相關方會被涉及到。企業管理者要么應該與他們直接溝通,要么應該讓同事們告訴他們將要發生什么。很多團隊的決策取決于大家的共識,因此,企業有必要想方設法減少來自利益相關方的阻力,避免部署被拖延。
接下來,公司需要預算足夠的時間來恰當地實施人工智能。他們必須花足夠的時間來理解利益相關者的角色和信念,評估有多少角色將會改變,并向人們解釋人工智能實際上會做什么,以及這個系統如何讓他們受益。
在人工智能技術的部署過程中,讓利益相關方放心也是很重要的。現在很多人仍然對人工智能技術懷有嚴重的恐懼、不確定和疑慮心理。企業可以就人工智能技術開展一些基礎性的教育培訓,以打消人們的這種疑慮。另外,企業還可以對人工智能系統進行嚴格的測試和審計,并且將結果展示給利益相關者,好讓他們相信,這項技術可以安全地工作。
另外,企業在部署人工智能技術時,可以先考慮進行試點,這樣的話,一開始只會有少數利益相關者受到影響。一旦試點項目迅速取得了成功,那它就更容易獲得大家的支持。
據普華永道估計,到2030年,全球人工智能技術的產值將達到15.7萬億美元,其中大部分產值將來自硅谷以外。人工智能即將改變每一個行業。如果企業在這個過程中采取了正確的做法,那么他們向人工智能轉型的過程就會容易得多。(財富中文網)
本文作者吳恩達(Andrew Ng)是Landing AI公司的創始人、CEO。
譯者:隋遠洙
眼下是一個人人都在談論人工智能的時代,但是要想把我們新聞里看到的那些似乎很有前景的試驗轉化成有實用價值的應用,卻還是得先搞定一些很重要的工作。大型的消費型互聯網公司是第一批實際應用人工智能的企業,但他們的程序未必適合其他行業,尤其是在很多行業中,人工智能項目都面臨著一些獨特的挑戰。
因此,我們經常會看到一些非數字型企業在部署人工智能項目時遇到困難的情況。比如制造業已經做好了向人工智轉型的準備,不過MAPI基金曾對美國200多家制造業企業進行過調查,其中只有5%的受訪企業制定了清晰的人工智能戰略。埃森哲公司也曾對16個行業的1500多名高管進行過調查,有76%的受訪者表示,他們在如何促進人工智能技術的規模化應用上遇到了困難。這種現象與消費型互聯網產業形成了對比,因為在這個產業中,大型的人工智能系統已經被應用到了從生成搜索結果,到語言翻譯,再到定向廣告的各個領域。
要想充分發揮人工智能技術的潛力,人工智能技術的開發者就必須研發一些新技術,確保人工智能在所有產業得到應用。(我的公司Landing AI就是一家專門幫助企業采用人工智能技術的公司。)尤其是對于硅谷以外的企業來說,他們必須要克服三個挑戰,才能增加成功的幾率。
首先,他們必須學會利用“小數據”。一些大型科技公司會使用從幾十億用戶那里收集到的海量數據來對人工智能模型進行訓練。但是很多行業沒有那么多的數據量,所以這些針對“大數據”研發的系統必須相應地做出一些調整,以適應這些行業的“小數據”。
比如有些工廠需要利用人工智能系統,檢測智能手機表面的劃痕。沒有哪家手機廠商會有100萬部有劃痕的手機放在那里,讓人工智能系統學習劃痕長什么樣子。所以說,很多制造企業是沒有足夠的數據來支撐人工智能模型的。制造業的人工智能軟件開發者,很多時候只能拿到不到100張的樣本照片來學習。
好在一種新的“小數據”技術正在使這一切成為可能。比如現在有了一種新的數據生成技術,它只需要10張高質量的瑕疵圖片,就可以合成1000張瑕疵圖片,供人工智能系統進行學習。除此之外還有一種方法,我們可以準備1萬張來自其他產品和數據來源的瑕疵圖片,人工智能模型先學習如何從這1萬張圖片中找出瑕疵品,了解了瑕疵品的一般規律后,它就可以把這一知識應用到一種新產品上,這時只需要額外的少量瑕疵圖片,它就可以識別出這種新產品的瑕疵了。
有了這種先進的“小數據”技術,人工智能很有可以最終得以打入制造業、農業和醫療等傳統行業。
其次,實驗室里的設定與真實世界是有很大差距的,如果人工智能技術的服務對象是非數字型企業,那么就要格外重視彌合這種差距。很多人工智能系統在研究論文里或者在概念驗證中展現了很高的準確性,但在真實世界部署時,其表現就沒有這么好了。
比如說,很多研究機構都發表論文稱,人工智能技術可以通過X光或者其他醫學影像來論斷病情,準確度堪比甚至超過了職業的放射科醫師。那么,為什么直到現在,各個醫院還是很少使用這項技術呢?
其中的一個原因是,很多此類研究的基礎設定都被控制得很好,人工智能模型的學習和測試對象都是高質量的數據。人工智能模型在這樣的設定中表現得很好,就足以做概念驗證或者發表論文了。不過如果同樣的一套人工智能系統被部署在了一家真正的醫院里,只要這家醫院的X光影像稍微模糊一點,或者它的圖像采集方案稍微有一些不一樣,這套系統就適應不了了。
對于這種情況,有一種解決方案是,一開始只用人工智能系統來分析它信心度比較高的圖像,其他情況都由人類放射科醫生來解決。然后讓人工智能系統向人類醫生學習,從而逐漸承擔更多的職能。
第三,對于一些非科技企業來說,他們有必要意識到,人工智能系統的部署,很可能影響到一些員工、客戶以及其他利益相關者的利益,所以必須妥善應對好這項新技術帶來的改變。
比如說,有一種人工智能系統是專門用來幫醫生對急診室的病人進行分類的,它的應用可能會影響到很多人——比如醫生、護士乃至保險業者。為了讓項目保持正軌,人們必須積極參與人工智能技術的部署當中。而為了充分利用這項新技術,他們的工作流程也必須做出相應的調整。
我發現,很多人工智能團隊都低估了組織變革管理中的人性化的一面。要想克服這個挑戰,并不是一件容易的事。但企業卻可以采取一些措施來減輕這種影響。
首先,企業必須認識到在變革的過程中,有哪些利益相關方會被涉及到。企業管理者要么應該與他們直接溝通,要么應該讓同事們告訴他們將要發生什么。很多團隊的決策取決于大家的共識,因此,企業有必要想方設法減少來自利益相關方的阻力,避免部署被拖延。
接下來,公司需要預算足夠的時間來恰當地實施人工智能。他們必須花足夠的時間來理解利益相關者的角色和信念,評估有多少角色將會改變,并向人們解釋人工智能實際上會做什么,以及這個系統如何讓他們受益。
在人工智能技術的部署過程中,讓利益相關方放心也是很重要的。現在很多人仍然對人工智能技術懷有嚴重的恐懼、不確定和疑慮心理。企業可以就人工智能技術開展一些基礎性的教育培訓,以打消人們的這種疑慮。另外,企業還可以對人工智能系統進行嚴格的測試和審計,并且將結果展示給利益相關者,好讓他們相信,這項技術可以安全地工作。
另外,企業在部署人工智能技術時,可以先考慮進行試點,這樣的話,一開始只會有少數利益相關者受到影響。一旦試點項目迅速取得了成功,那它就更容易獲得大家的支持。
據普華永道估計,到2030年,全球人工智能技術的產值將達到15.7萬億美元,其中大部分產值將來自硅谷以外。人工智能即將改變每一個行業。如果企業在這個過程中采取了正確的做法,那么他們向人工智能轉型的過程就會容易得多。(財富中文網)
本文作者吳恩達(Andrew Ng)是Landing AI公司的創始人、CEO。
譯者:隋遠洙
While artificial intelligence has become a ubiquitous topic in the business world, there is still important work to do to translate the promising experiments we see in the news to valuable and practical implementation. Large consumer Internet companies pioneered practical A.I. deployments, but their processes do not necessarily apply in other industries where A.I. projects face unique challenges.
As a result, we frequently see non-digital companies struggle with A.I. deployment. Manufacturing, for instance, is primed for A.I. transformation, but only 5% of more than 200 manufacturers surveyed by the MAPI Foundation say they have a clearly defined strategy for A.I. In a separate Accenture report that surveyed 1,500 C-suite executives in 16 industries, 76% of respondents said they struggle with how to scale the technology. This stands in contrast to the consumer Internet industry, where large A.I. systems already power everything from producing search results to language translation to targeted advertising.
For A.I. to reach its full potential, those implementing the technology must develop new techniques to enable its deployment across all industries. (My company, Landing AI, helps companies with A.I. adoption.) In particular, companies outside Silicon Valley need to overcome three challenges to increase their odds of success.
First, they must learn to harness small data. The tech giants use vast volumes of data collected from billions of users to train A.I. models. Techniques developed for these big data settings need to be adapted to the much smaller datasets that most other industries have.
Take the challenge of building an A.I.-powered system for a factory to detect scratches on smartphones. No smartphone manufacturer has a million scratched phones lying around from which it can capture pictures of scratches. Thus, many manufacturers do not have enough data to power conventional A.I. models. Manufacturing A.I. application builders often need to get by with 100 or fewer images.
Fortunately, new small data technologies are starting to make this possible. For example, a new data generation technique may be able to take 10 images of a rare defect and synthesize an additional 1,000 images that an A.I. system can then learn from. Using another method, an A.I. model might first learn to find dents from a large dataset of 10,000 pictures of dents collected from different products and data sources. Having learned about dents in general, it can then transfer this knowledge to detect dents in a specific novel product with only a few pictures of dents.
Such advanced small data techniques may enable A.I. to finally break into traditional industries like manufacturing, agriculture, and health care.
Second, A.I. models serving non-digital firms must bridge the gap between research settings and the real world. Many A.I. systems that achieve high accuracy in a research paper or proof of concept do not perform as well when deployed.
For example, many research groups have published articles that report A.I.’s ability to diagnose from x-rays or other medical images at a level of accuracy comparable or superior to that of radiologists. So why is it still so rarely used?
One reason is that many of these studies are carried out in well-controlled settings where the A.I. learns from and is tested on consistently high-quality data. Doing well in such a setting leads to a successful proof of concept or publication. However, if the same A.I. system is deployed in a hospital where x-ray images are slightly blurrier or the protocol for collecting images is slightly different, it fails to adapt.
One solution is to start by only using A.I. to analyze images on which it has high confidence, while relying on a human radiologist for all other cases. The A.I. then learns from the radiologist and is gradually able to take on more responsibility.
Third, non-tech companies deploying A.I. must be aware of its potential to disrupt employees, customers, and other stakeholders in the business, and appropriately manage the change the technology brings.
For instance, an A.I. system that helps doctors triage patients in an emergency room affects many—from doctors and intake nurses to insurance underwriters. To keep projects on track, people must be brought on board with A.I. implementation, and their workflow must be adjusted to take advantage of the technology.
I have seen many A.I. teams underestimate the human side of organizational change management. Overcoming this challenge is not easy, but there are steps businesses can take to mitigate disruption.
For one, organizations have to identify all the stakeholders that will be involved with the change process. Managers should either communicate with them directly or find ways to have their colleagues talk to them about what is coming. Many teams make decisions by consensus, so it is important to minimize the odds of any stakeholder blocking or slowing down implementation.
Next, companies need to budget enough time to properly implement A.I. They must spend enough time to understand stakeholders’ roles and beliefs, assess how many roles will change, and explain to people what the A.I. will actually do and how the system may benefit them.
It’s crucial that the company reassure stakeholders during A.I. implementation. Many people still harbor significant fear, uncertainty, and doubt about A.I. Providing a basic education about the technology eases these conversations. Organizations can also reassure people by rigorously testing and auditing the technology, and showing the results to stakeholders so they’re convinced it works safely.
Organizations should consider beginning A.I. deployment with a pilot that affects a relatively small number of stakeholders. A quick success can then be used as a showcase to get buy-in from a larger group.
PwC estimates that A.I. will generate $15.7 trillion globally by 2030. Much of this value will come from outside Silicon Valley. A.I. is on its way to transforming every industry; the process will be a lot easier if businesses take the right actions along the way.
Andrew Ng is founder and CEO of Landing AI.